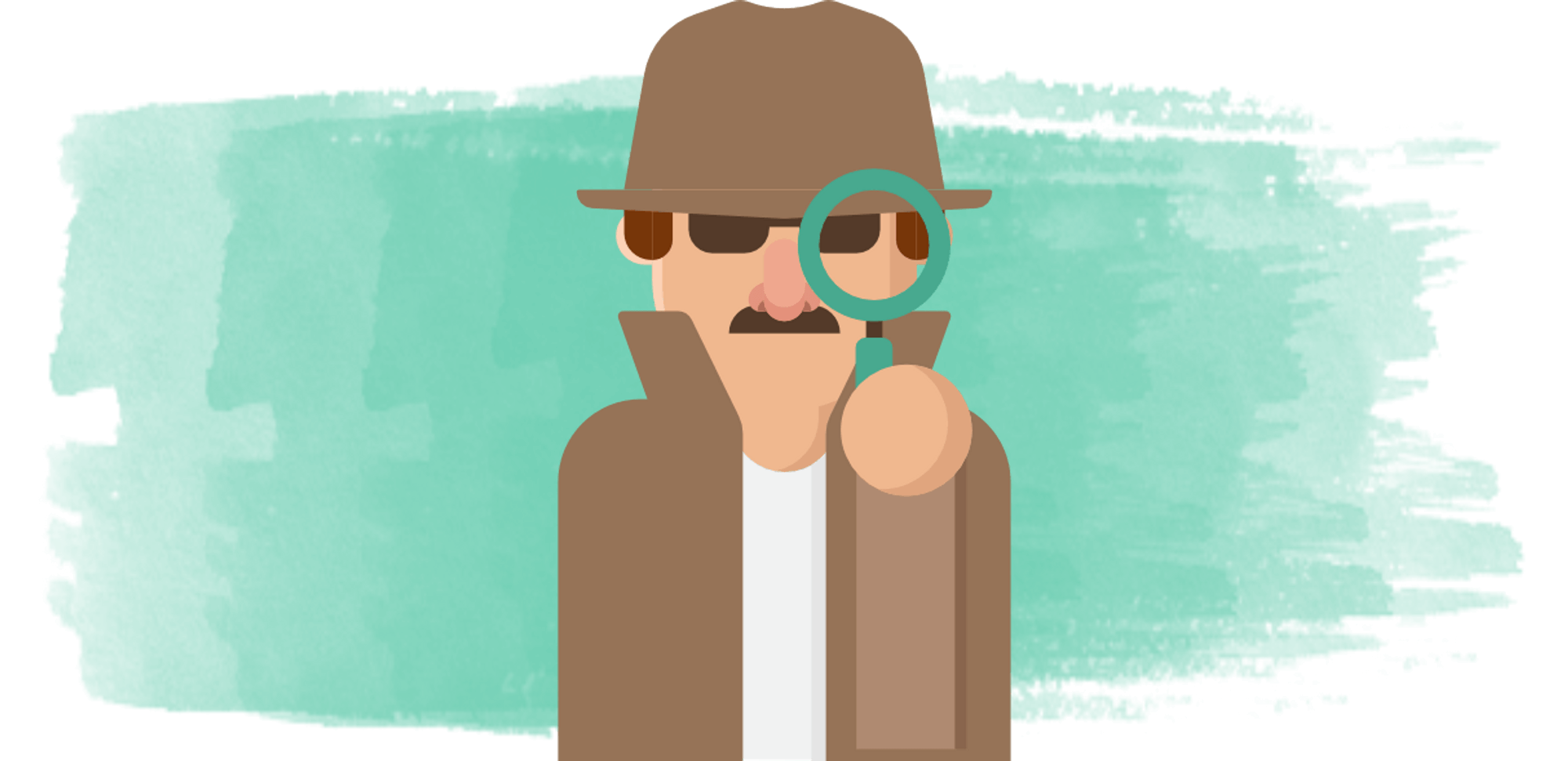
Statistical Comparison and Significance
“Quantitative research is great for determining the scale or priority of design problems, benchmarking the experience, or comparing different design alternatives in an experimental way.” [1]
- We recommend performing A/B testing, for example using groups with and without transparency-communication, to better understand the results, see how much they differ and if their difference is significant.
- For statistical comparison of differential hypotheses – comparing the results of two or more groups – we recommend the following steps to find the correct statistical method:
💡 To calculate the statistical significance with the online calculators, paste all raw-data of every tester into the calculators. E.g. all UEQ-S likert scale answers from testers of group 1 into the first column and all data from group 2 into the second column.
To know which statistical methods to use you have to check your data for normal distribution first and see if they are interval-scaled.
If your data is normally distributed and interval-scaled you should use parametric tests. We recommend:
t-test for two dependent or independent samples
ANOVA for three or more samples (dependent or independent)
💡 Dependent samples are from the same set of individuals. Independent samples are from different sets of individuals.
If your data is not normally distributed or not metric you should use non-parametric tests. We recommend:
Wilcoxon Signed Rank test for dependent samples
Mann-Whitney test for independent
samples
💡 It depends on your hypothesis if you have to use one-tailed or two-tailed testing [3]:
- One-tailed testing is used for a directional hypothesis. For example: “Using light as feedback modality will increase the feeling of control compared to no feedback.”
- Two-tailed testing is used for a non-directional hypothesis. For example: “There is a difference in the feeling of control regarding light as a feedback modality and no feedback.”
Statistical Significance
When using statistical methods for your hypotheses you want their results to be significant at p<.05 or even p<.01.
"Statistical significance" refers to the probability that the observed result could have occurred randomly if it has no true underlying effect. This probability is usually referred to as "p" and by convention, p should be smaller than 5% to consider a finding significant.
Sometimes researchers insist on stronger significance and want p to be smaller than 1%, or even 0.1%, before they'll accept a finding with wide-reaching consequences, say, for a new blood-pressure medication to be taken by millions of patients.” [2]
Sources
[1] - Moran, Kate. "Quantitative Research: Study Guide" URL: https://www.nngroup.com/articles/quantitative-research-study-guide/[Accessed September 2022] 8 (2021).
[2] - Nielsen, Jakob. "Understanding Statistical Significance" URL: http://www.nngroup.com/articles/usability-101-introduction-to-usability/[Accessed September 2022] 3 (2014).
[3] - Surbhi, S. "Difference Between One-tailed and Two-tailed Test" URL: https://keydifferences.com/difference-between-one-tailed-and-two-tailed-test.html[Accessed September 2022] 2 (2018).